Artificial intelligence AI is set to revolutionize 77% of businesses that are either using or looking forward to implementing it. The increasing adoption of AI into all industries and business processes is altering every walk of life. Statista predicts that AI’s market value will soar to $100 billion by 2030.
However, AI is only as good as the data it learns from. For machine learning systems to perform well, an enormous amount of training data must be fed to these models to be able to provide high-quality outputs.
Imagine a self-driving car navigating a busy street with limited knowledge of traffic signals or pedestrians. The consequences could be disastrous. And that’s why data annotation is crucial.
So, what is data annotation, why does it matter, and what does it add to the field of localization? You will find out about this and more in this blog.
Let’s get into it!
What is Data Annotation? A Closer Look at the Data Annotation Process
Data annotation is the process of labeling or tagging raw visual data with descriptive or identifying information to enable machine learning models to comprehend and classify information, recognize patterns, and “see” and interpret the world around them. These data could be images, video, text, audio, and more.
To unlock your mobile with your face, for example, your mobile should be able to recognize visual input. It is through the power of data annotation that your mobile is taught to identify objects, patterns, and other information.
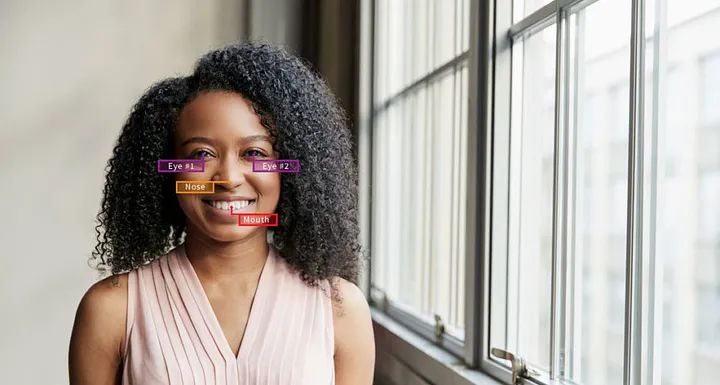
Types of Data Annotation
It’s impossible for AI systems to function well without quality data. There are many forms of data annotation, depending on the requirements of the machine learning task and the type of data.
- Image Annotation
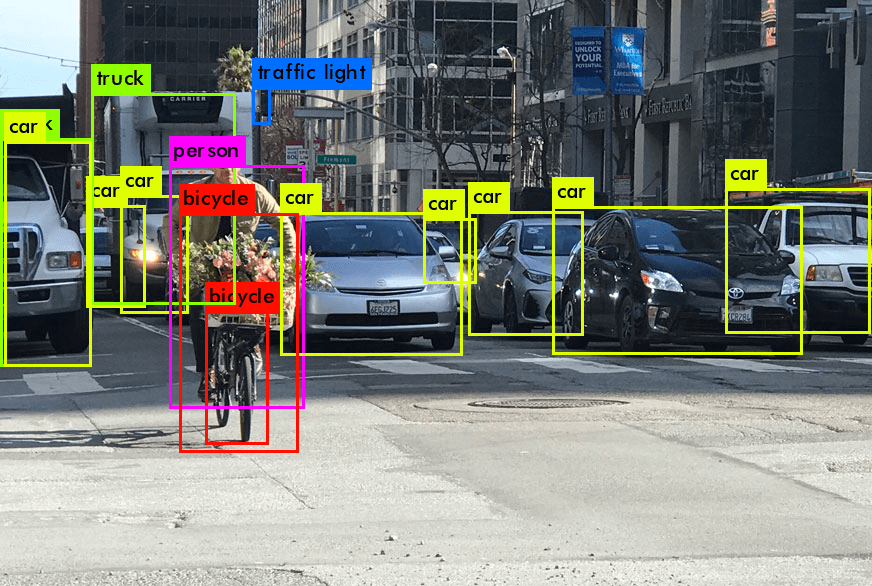
Image annotation is the process of labeling images with metadata to assist AI systems in object detection and recognizing scenes and other visual elements.
Image annotation is crucial in various AI-based applications, such as self-driving cars (for object detection), facial recognition (for identifying features), and computer vision (for scene understanding).
Common Types of Image Annotation:
- Bounding Boxes
A bounding box, also known as a bounding region or bounding volume, is an imaginary rectangle shape that surrounds one object or a group of objects.
Data annotation teams draw these geometric shapes over machine learning images to outline objects, defining the location and size of this exact object. This will enable machine-learning algorithms to detect the object and determine collision paths.
- Semantic Segmentation
Semantic segmentation is a task that labels each pixel of an image with an object or class and classifies them depending on various characteristics.
Its main target is to enable machine learning algorithms to comprehend and process visual information. It’s one of the integral tasks in many AI applications, such as robotics, medical image analysis, and autonomous driving.
- Keypoints Annotation
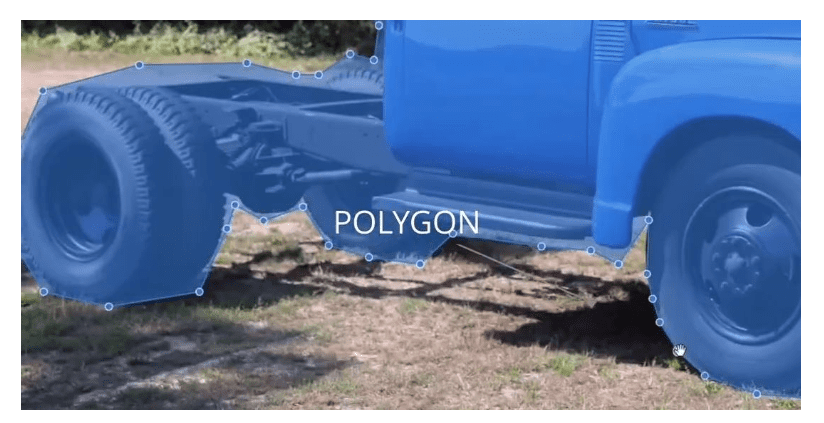
Keypoints annotation is the process of marking specific points of interest to track variations between objects, such as human figures.
Points can capture the exact details of a human figure, like the head, shoulders, knees, etc. This can provide data about the figure’s structure, movement, and pose.
- Polygon Annotation
Polygon annotation is a technique that better handles irregular shapes by drawing multi-sided shapes over the object of interest.
The Polygon tool is more precise than the bounding box due to its ability to capture high levels of detail.
- Video Annotation
Video annotation is the process of labeling video frames with metadata to track objects, actions, and events over time. As one of the main tasks for applications like surveillance, autonomous driving, and object tracking, video annotation feeds AI models with the needed data to analyze movements and track objects.
Some common types of video annotation:
- Object Tracking
Object detection and tracking in videos is a technique that involves identifying and tracing a specific object as it moves through consecutive frames of a video.
Annotators label the object of interest in each frame, enabling AI models to monitor and analyze movement patterns, identify anomalies, and understand object interactions within a video feed.
- Action Recognition
Commonly used in sports, action recognition is the technique that identifies and categorizes different actions or activities performed by objects or humans in a video, such as walking, running, jumping, and more.
- Video Classification
Video classification is the process of categorizing and labeling videos based on their content, whether it’s comedy, action, drama, etc, by analyzing the visual and audio content.
It enables systems to understand and categorize videos for various applications such as content recommendation, video search, and automated video tagging.
- Text Annotation
With the many advances that AI has made, AI is still limited when the goal is to produce natural-sounding text. AI still struggles with sarcasm, idioms, and other contextual content.
A human processes the meaning of a statement after going through so many steps: understanding the meaning of words and then phrases, relating them to this exact situation. However, AI can’t dive to these deep levels, only scratching the surface.
In light of the above, text annotation interferes with training machine learning algorithms to comprehend and process textual information effectively by adding metadata, labels, or tags to raw text data.
Some of the common text annotation techniques are:
- Entity Recognition
Entity recognition is identifying and classifying named entities mentioned in unstructured text into predefined categories such as names of persons, organizations, locations, expressions of times, quantities, monetary values, percentages, etc.
- Semantic Annotation
Semantic annotation revolves around tagging concepts such as people, places, or company names within a document to help AI algorithms classify and categorize any new concepts, a process that is very crucial for Chatbots!
- Intent Annotation
Intent annotation helps AI models detect and understand the intention and purpose behind a user’s language. This is especially crucial for building chatbots, virtual assistants, and sentiment analysis models.
By annotating text with specific intents, such as asking a question, expressing satisfaction, or making a complaint, AI models can better understand and categorize the text, leading to more accurate and practical natural language understanding and processing.
- Text Classification
Text classification or categorization categorizes sentences and paragraphs into predefined classes, which makes it easy for an AI model to link text documents to specific topics, trends, and concepts.
- Audio Annotation
In order for AI algorithms to comprehend the language and behavior of a user and their intent and emotions, audio annotation is the only way to go. It’s the process of labeling audio data with metadata to identify sounds, speech, and other acoustic elements.
Audio annotation involves tagging and labeling not only verbal cues but also non-verbal cues, such as silence, background noise, and breaths.
Some of the common audio annotation techniques are:
- Audio Transcription
Audio transcription converts spoken language to written text in real time—a crucial technique for creating captions for films, TV shows, and interviews.
- Sound Classification
Sound classification is the technique Spotify uses to classify music genres and recommend relevant tracks to their users. It categorizes and labels audio clips based on their sound characteristics.
Especially useful in applications such as speech recognition, environmental sound monitoring, music analysis, and audio content recommendation systems, sound classification allows for identifying different sounds or audio patterns within the audio data, such as music, speech, ambient noise, or specific sound effects.
The Importance of Data Annotation in AI and Localization
Now, how does data annotation serve the field of localization?
is very important in AI-driven localization. It ensures that digital content is accurately adapted to diverse audiences. By refining AI models through precise data labeling, companies can deliver more culturally relevant and personalized experiences, making localization efforts more efficient and impactful.
So, how does data annotation serve the field of localization? Let’s break it down:
1. Training AI for Accurate and Nuanced Translation
Imagine trying to teach a child a new language by simply handing them a dictionary. They might learn individual words, but understanding context, tone, and cultural idioms would be a challenge. AI faces a similar challenge.
Data annotation provides the context AI needs to truly “learn” a language. By tagging text with information like:
- Part of speech: Is the word a noun, verb, or adjective?
- Sentiment: Is the tone positive, negative, or neutral?
- Named entities: Are there people, places, or organizations mentioned?
This allows AI to move beyond literal translations and capture the subtleties of human language, ensuring more nuanced and accurate results.
2. Adapting Content for Cultural Relevance
Localization goes beyond translation; it’s about making content resonate with users from different cultures. With the power of data annotation, we can label phrases, idioms, and expressions specific to certain cultures or regions and utilize AI for tailoring visual content to particular demographics.
- Adjusting images and icons: A thumbs-up might be a positive gesture in one culture but offensive in another.
- Adapting date and time formats: Different regions have different conventions for displaying dates and times.
- Customizing user interface elements: The layout and flow of an app might need to be adjusted for different cultural preferences.
Labeling images, videos, and other content with cultural tags, teaches AI to recognize what’s appropriate and effective for different audiences.
For example, in the localization of marketing materials or user interfaces, entity recognition can help identify product names, brands, or culturally specific references that require special attention during translation.
3. Streamlining the Localization Workflow
Localization can be a complex and time-consuming process. Data annotation helps streamline this workflow by:
Automating repetitive tasks: AI can be trained to automatically translate certain phrases or adapt content formats, freeing up human translators to focus on more complex linguistic challenges.
Ensuring consistency: Using a centralized data annotation platform, companies can ensure consistency in terminology and style across all localized content. For example, it helps identify recurring terms or references that may have multiple variations in the target language.
4. Improved Customer Experience with Sentiment Analysis
Data annotation is transforming the eCommerce sector by facilitating better product categorization and personalization. It enables AI to:
- Understand Emotional Tone: Quickly assess customer feedback to gauge perceptions and align products with cultural preferences.
- Analyze Customer Behavior: Labeling data on customer interactions helps identify buying patterns and preferences, leading to targeted recommendations and increased sales.
- Efficient Customer Service
Data annotation supports efficient customer service by:
- Classifying Inquiries: AI models trained with annotated data can accurately categorize customer messages (e.g., billing, technical support, product information) and route them to the appropriate department.
- Enhancing Automation: Natural Language Processing (NLP) models use tagged data to understand customer intent, automating responses and resolutions more effectively.
In the end, as AI continues to revolutionize various industries, the emphasis on accurate and comprehensive data annotation will be paramount in realizing the true transformative power of artificial intelligence.
Data annotation unlocks the full potential of AI for businesses to thrive globally, enabling them to optimize their operations and get well-performing AI models.
Unlock the full potential of AI with AsiaLocalize!
Get Started Now!